By Prof Mu Koh, Royal Marsden NHS Foundation Trust (ICR)
Primary prostate cancer is a heterogeneous disease in terms of disease aggressiveness and long-term outcomes. Many men who are diagnosed with prostate cancer will have indolent disease and die of other causes. However, some men have aggressive disease that results in cancer spreading to other parts of the body, particularly the bones. Approximately 50% of men diagnosed with local prostate cancer will develop metastases in their lifetime. In particular, bone metastasis is associated with significant morbidity including bone pain, pathological fracture, and spinal cord compression. Hence, confident identification of patients at risk for early metastatic disease can influence diagnostic, surveillance, and treatment strategies to improve patient outcomes.
Different multivariate models and nomograms have been proposed to identify the risk of cancer progression based on clinical and laboratory data. These include parameters such as the serum PSA levels, the Gleason score of the primary tumour at biopsy, patient age at diagnosis, clinical tumour stage, and the percentage of biopsy core samples positive for cancer. However, many of these models have been developed in the context of biochemical recurrence, usually after treatment. Nonetheless, using the CAPRA score, it has been shown that each point increase in the score was associated with an increased risk of bone metastases (HR for metastasis = 1.47, 95% CI = 1.31. to 1.48) and increased risk of mortality.
The use of artificial intelligence and machine learning in primary prostate cancer has the potential to uncover novel imaging features that inform upon the likelihood of early metastatic disease. Prior studies using traditional radiomics methods on prostate MRI have achieved good diagnostic performance (AUC 0.76 to 0.93) for identifying patients with nodal or bone metastases. However, the potential to use more advanced machine learning techniques and deep radiomics methods to uncover novel meaningful signatures has not been established. In the ProCAncer-I study, we will use our well-curated multicentre imaging dataset to extend current knowledge by applying advanced artificial intelligence and machine learning techniques to identify patients with a high likelihood of developing early metastatic disease. We will evaluate standard clinical and radiological features alone and in combination with our imaging models to identify early metastatic disease. Patients who have developed metastases in the first year of diagnosis will be the positive class in our model, while patients without metastases will be the negative class.
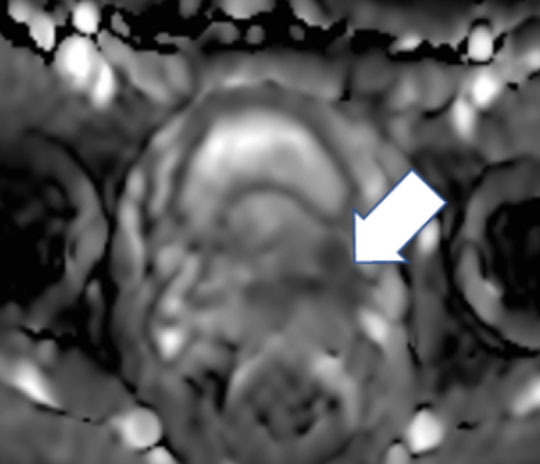
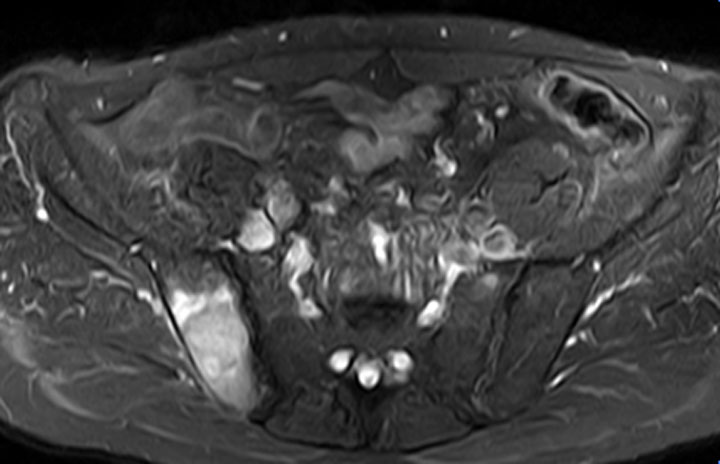
A 57 year-old man with a PI-RADS 5 lesion at the left base of the prostate gland shown here on the ADC map (arrow).
STIR MR imaging shows a synchronous bone metastasis within the right ilium.