- Patient-level diagnosis or lesion-level classification (CADx)
- Lesion-level detection (CADe)
CADx describes algorithms for the classification of lesion candidates on MRI. They are primarily used to classify manually-annotated lesion candidates in two or multiple classes. Lesion-level CADx algorithms are semi-automated, i.e. readers are still required to manually annotate lesions candidates for further characterization, which does not reduce the current workload for prostate MRI assessment.
A different approach is that of lesion detection algorithms or CADe. Its pipeline is shown in Fig. 1. In comparison to CADx, CADe algorithms do not require manual input of lesion candidates or a region-of-interest, as images are classified on an object or voxel-level basis. For this reason, PCa detection algorithms could aid in prostate MRI assessment, by automatically highlighting suspicious regions with cancer likelihood maps and/or segmentations to the reader, which in turn, could decrease the current workload of radiologists.
Recent literature has described multiple algorithms for this task with varying results. Additionally, in the current landscape of AI developments for PCa, much of the AI work is limited to single-center, single AI-architecture analyses, and critically, on small data sets.
The scope of UC 1: “Detection of prostate cancer with high accuracy in both the peripheral and transitional zone” is to improve the accuracy of PCa detection on mpMRI, powered by AI models trained on a large collection of multicenter mpMRI exams. It serves as a first step in the addressment of the current unmet clinical needs in PCa regarding precision diagnosis and personalized disease management while it provides lesion candidates open for further characterization. Within this UC, AI-models will be developed, classifying lesions candidates as cancerous or non-cancerous. Models will be trained on mpMRI images combined with clinical data such as PSA, PSAD and or follow-up. In total 13.000 cases will be dedicated to this clinical question.
Figures and text are excerpts from the recent ProCancer-I funded narrative review:
Twilt JJ, van Leeuwen KG, Huisman HJ, Fütterer JJ, de Rooij M. Artificial Intelligence Based Algorithms for Prostate Cancer Classification and Detection on Magnetic Resonance Imaging: A Narrative Review. Diagnostics. 2021; 11(6):959. https://doi.org/10.3390/diagnostics11060959
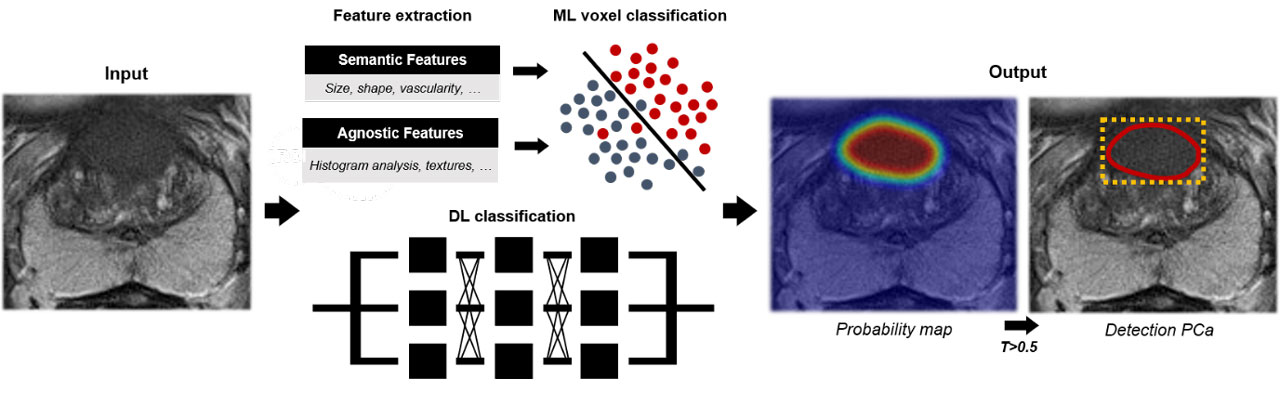